Statistical Techniques on Nonlinear Manifolds -- Their Contributions in Advancing Image Understanding
Prof. Anuj Srivastava
Department of Statistics, Florida State University
http://stat.fsu.edu/~anuj
Abstract:
The primary goal in image understanding is to characterize objects contained in images, in terms of their locations, motions, activities, and appearances. Due to inherent variability associated with scenes, images, and objects, statistical approaches becomes natural. Any statistical approach requires mathematical representations of objects of interest and probabilistic descriptions to capture their variabilities. The difficulty comes from nonlinearity of object representation spaces -- these are not Euclidean and one cannot perform classical multivariate statistics directly. Instead, one needs tools from differential geometry, for handling the nonlinearity, and statistical techniques adapted to these manifolds for performing object characterization. In contrast to manifold-learning problems where one estimates the underlying manifold, the representation spaces here are fully known and one need to exploit their geometries to develop efficient statistical tools.
An example of this situation arises in shape analysis of objects in still images and videos. While shapes have been represented in many ways -- point sets, level-sets, parametrized curves, boundary surfaces, etc -- their representation spaces mostly form nonlinear manifolds. Here one would like to compare shapes, average them, develop statistical shape models, and provide tests for hypotheses involving different shape classes, and the nonlinearity of shape manifolds presents a challenge. I will describe recent advances in differential-geometric techniques that overcome this challenge and provide a rich set of techniques for "elastic shape analysis." The resulting tools include computation of distances for joint shape registration and comparisons, averaging of shapes, principal component analysis to discover modes of variations, "Gaussian"-type shape models, and much more. This framework helps capture shape variability in datasets very efficiently using low-dimensional manifolds and corresponding statistical models. This approach has been applied to shape analysis in face recognition, activity classification, object recognition, medical diagnosis, and bioinformatics.
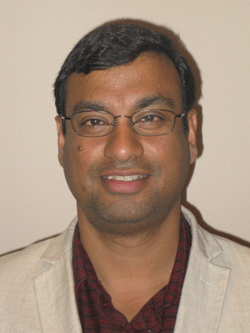
Biography:
Anuj Srivastava is a Professor in the Department of Statistics at the Florida State University in Tallahassee, FL. He obtained his PhD degree in Electrical Engineering from Washington University in St. Louis in 1996 and was a postdoc at Division of Applied Mathematics at Brown University during 1996-1997. He joined the Department of Statistics at the Florida State University in 1997 as an Assistant Professor. Subsequently, he was promoted to Associate Professor in 2003 and to full Professor in 2007. He has held visiting positions at INRIA, Sophia Antipolis, France; Université Catholique de Louvain, Belgium; and University of Lille, France. He is also a recipient of the Durham International Senior Fellowship from Durham University in UK for 2014. His areas of research include statistics on nonlinear manifolds, statistical image understanding, functional data analysis, and statistical shape analysis. He has published more than 180 papers in refereed journals and proceedings of refereed international conferences. He has been an associate editor for Journal of Statistical Planning and Inference, IEEE Transactions on Signal Processing, and IEEE Transactions on Pattern Analysis and Machine Intelligence.